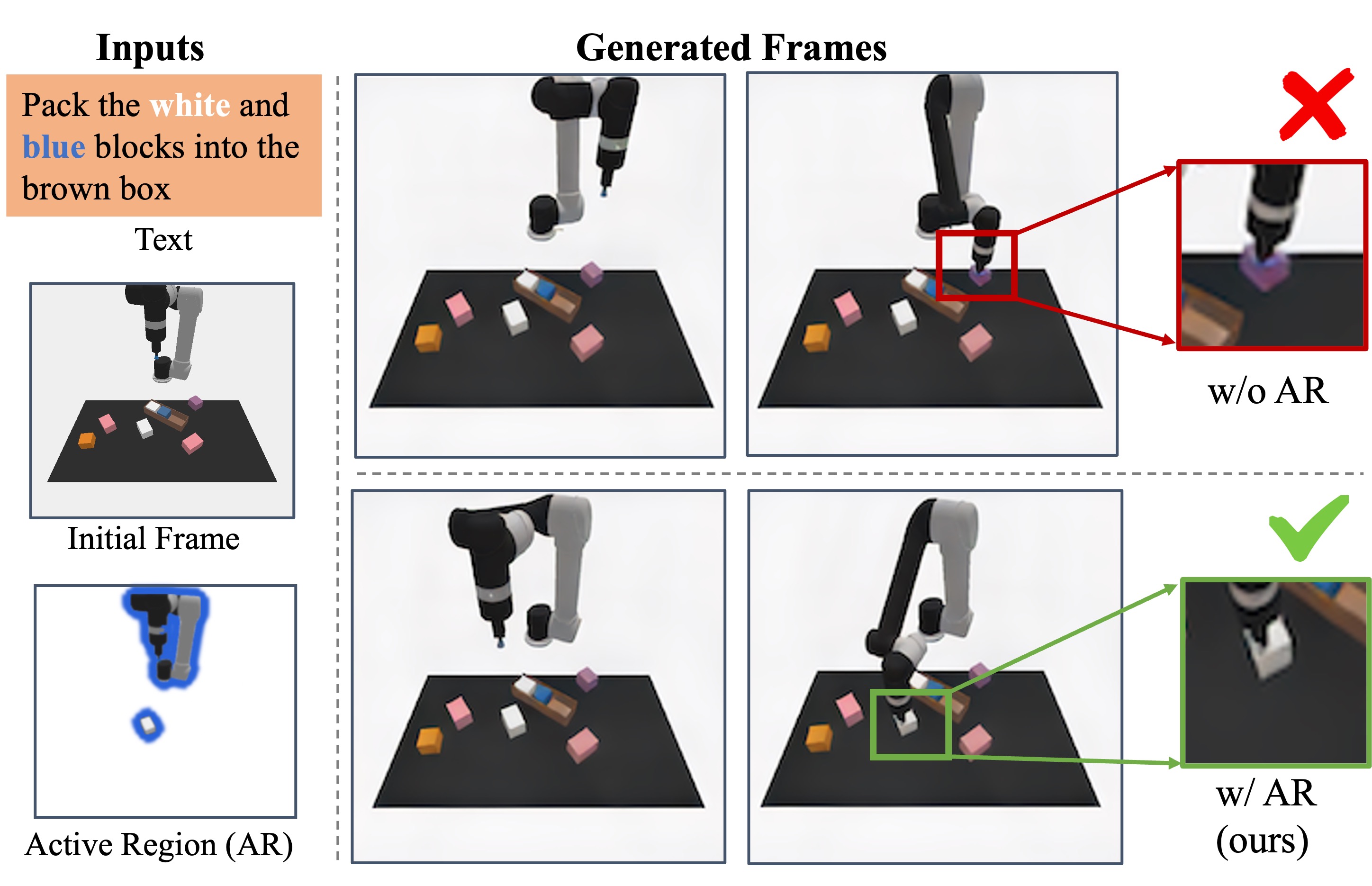
Sequential decision-making can be formulated as a text-conditioned video generation problem, where a video planner, guided by a text-defined goal, generates future frames visualizing planned actions, from which control actions are subsequently derived. In this work, we introduce Active Region Video Diffusion for Universal Policies (ARDuP), a novel framework for video-based policy learning that emphasizes the generation of active regions, i.e. potential interaction areas, enhancing the conditional policy’s focus on interactive areas critical for task execution. This innovative framework integrates active region conditioning with latent diffusion models for video planning and employs latent representations for direct action decoding during inverse dynamic modeling. By utilizing motion cues in videos for automatic active region discovery, our method eliminates the need for manual annotations of active regions. We validate ARDuP’s efficacy via extensive experiments on simulator CLIPort and the real-world dataset BridgeData v2, achieving notable improvements in success rates and generating convincingly realistic video plans.