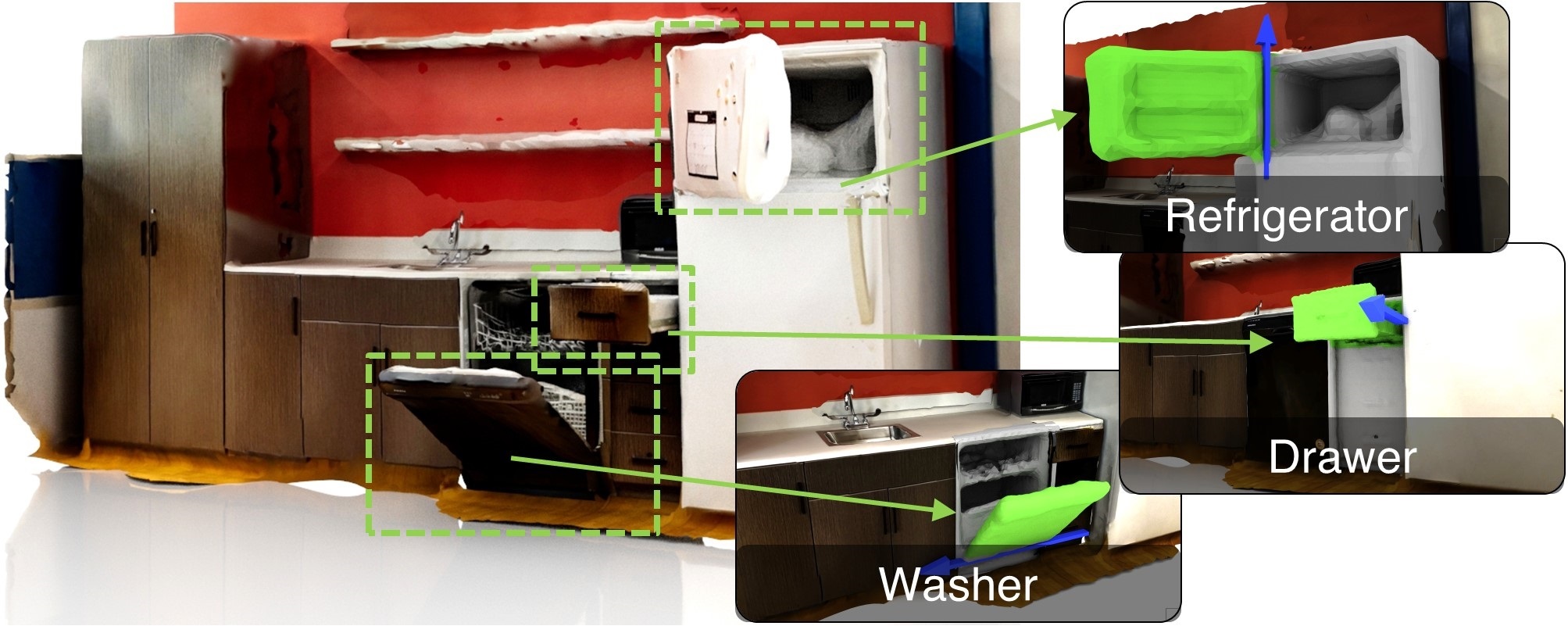
Virtualizing the physical world into virtual models has been a critical technique for robot navigation and planning in the real world. To foster manipulation with articulated objects in everyday life, this work explores building articulation models of indoor scenes through a robot’s purposeful interactions in these scenes. Prior work on articulation reasoning primarily focuses on siloed objects of limited categories. To extend to room-scale environments, the robot has to efficiently and effectively explore a large-scale 3D space, locate articulated objects, and infer their articulations. We introduce an interactive perception approach to this task. Our approach, named Ditto in the House, discovers possible articulated objects through affordance prediction, interacts with these objects to produce articulated motions, and infers the articulation properties from the visual observations before and after each interaction. It tightly couples affordance prediction and articulation inference to improve both tasks. We demonstrate the effectiveness of our approach in both simulation and real-world scenes. Code and additional results are available at https://ut-austin-rpl.github.io/HouseDitto/