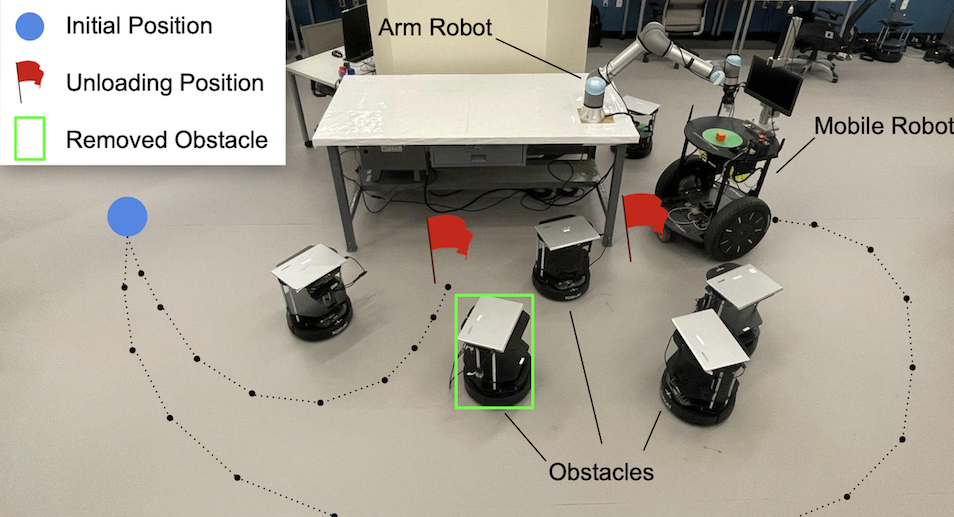
Task and motion planning (TAMP) algorithms aim to help robots achieve task-level goals, while maintaining motion-level feasibility. This paper focuses on TAMP domains that involve robot behaviors that take extended periods of time (e.g., long-distance navigation). In this paper, we develop a visual grounding approach to help robots probabilistically evaluate action feasibility, and introduce a TAMP algorithm, called GROP, that optimizes both feasibility and efficiency. We have collected a dataset that includes 96,000 simulated trials of a robot conducting mobile manipulation tasks, and then used the dataset to learn to ground symbolic spatial relationships for action feasibility evaluation. Compared with competitive TAMP baselines, GROP exhibited a higher task-completion rate while maintaining lower or comparable action costs. In addition to these extensive experiments in simulation, GROP is fully implemented and tested on a real robot system.