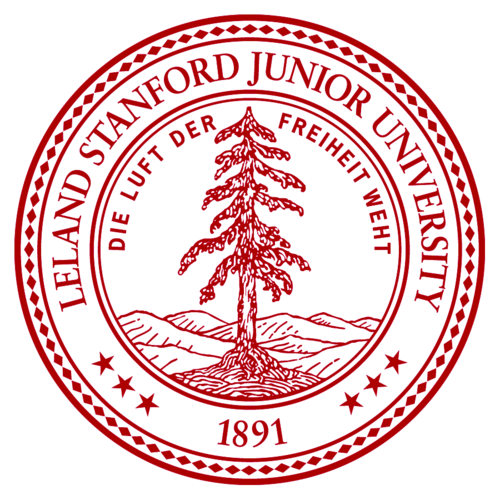
Robots and autonomous systems have been playing a significant role in the modern economy. Custom-built robots have remarkably improved productivity, operational safety, and product quality. However, these robots are usually programmed for specific tasks in narrow domains, unable to quickly adapt to new tasks and novel situations. The advent of affordable, lightweight, and flexible robot hardware has opened up opportunities for scaling up robot autonomy to an unprecedented level. A major challenge for the new robot hardware to operate in everyday settings is to handle the constant variability and uncertainty of the real world. To tackle this challenge, we have to address the synergy between perception and action: on the one hand, the robot’s perception guides its action adaptively, and on the other hand, its action gives rise to new perceptual information for decision making. I argue that a vital step towards a general-purpose robot autonomy is to integrate perception and action in a tight loop. Emerging computational tools in artificial intelligence have demonstrated promising successes and constitute ideal candidates to enhance robots’ perception and control in unstructured environments. The embodied nature of robotics compels us to move beyond the existing paradigm of learning from disembodied datasets and inspires us to develop novel algorithms that take into account the physical hardware and the complex system dynamics. This dissertation demonstrates our research that builds methods and mechanisms for generalizable robot perception and control. Our work illustrates that the tight coupling of perception and action facilitates robots to interact with the unstructured world through their senses, to flexibly perform a wide range of tasks, and to adaptively learn new tasks. Our findings show that dissecting the perception-action loop at three levels of abstraction, from the low-level motor skills to high-level task understanding, effectively prompts the robustness and generalization of robot behaviors. Laying out our research work that attends to tasks with growing complexity unfolds our roadmap towards the holy-grail goal: building long-term, general-purpose robot autonomy in the real world.