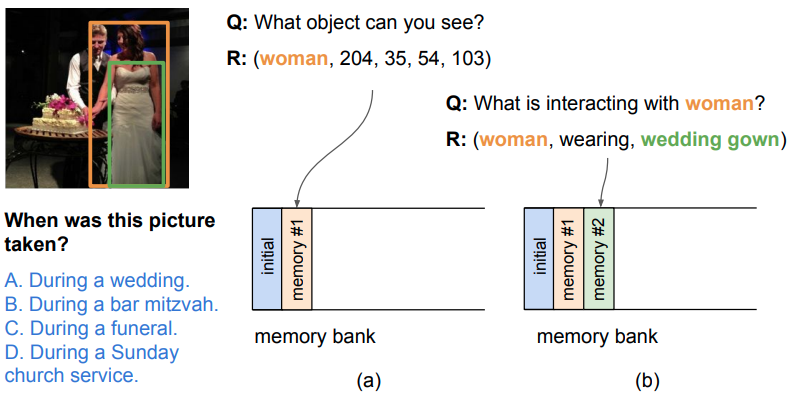
Humans possess an extraordinary ability to learn new skills and new knowledge for problem solving. Such learning ability is also required by an automatic model to deal with arbitrary, open-ended questions in the visual world. We propose a neural-based approach to acquiring taskdriven information for visual question answering (VQA). Our model proposes queries to actively acquire relevant information from external auxiliary data. Supporting evidence from either human-curated or automatic sources is encoded and stored into a memory bank. We show that acquiring task-driven evidence effectively improves model performance on both the Visual7W and VQA datasets; moreover, these queries offer certain level of interpretability in our iterative QA model.